The Role of Generative AI in Identifying Cyber Vulnerabilities
Share
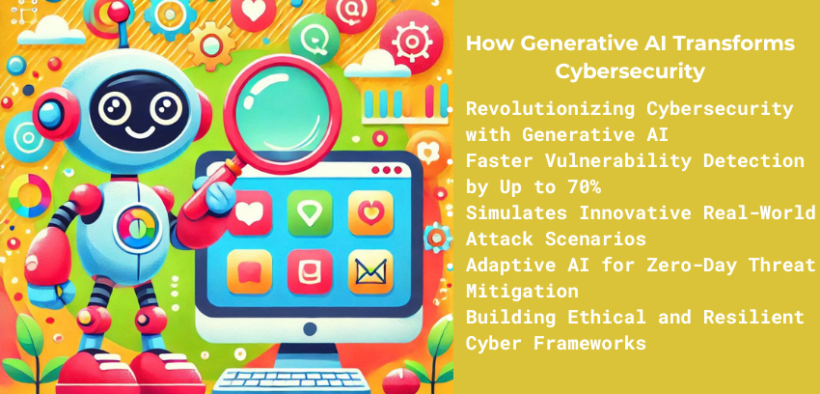
Generative AI is emerging as a transformative tool in identifying cyber vulnerabilities, offering innovative solutions that enhance cybersecurity efforts. With the rising sophistication of cyber threats, leveraging AI technologies has become essential for organizations to protect their digital assets effectively. This article delves into how Generative AI enhances vulnerability detection, simulates innovative attack vectors, and strengthens penetration testing workflows, providing practical insights for both seasoned and amateur cybersecurity professionals.
Understanding Generative AI in Cybersecurity
Generative AI, powered by Large Language Models (LLMs), is designed to mimic human reasoning, making it a powerful ally in cybersecurity. By automating complex tasks such as network scans and vulnerability analysis, Generative AI minimizes human effort while maximizing precision. Unlike traditional tools, it continuously learns from evolving cyber threats, ensuring adaptability and resilience.
Stat: The global cost of cybercrime is projected to hit $10.5 trillion by 2025, up from $8 trillion in 2023, making advanced tools like Generative AI indispensable for organizations. (Source: Hilario et al., 2024)
Detecting Cyber Vulnerabilities with Generative AI
Generative AI is redefining how cyber vulnerabilities are identified through:
- Automated Analysis: It scans networks, systems, and applications, uncovering hidden weaknesses.
- Data Correlation: By analyzing vast datasets, it identifies patterns and potential threats that traditional tools might miss.
- Continuous Updates: It evolves with emerging threats, maintaining relevance in detecting zero-day vulnerabilities.
Stat: AI-driven tools reduce reconnaissance and vulnerability scanning time by 50%-70%, significantly enhancing efficiency. (Source: Hilario et al., 2024)
Simulating Innovative Attack Vectors
One of Generative AI’s standout features is its ability to simulate complex attack scenarios that mirror real-world tactics:
- Unconventional Attack Vectors: Identifies vulnerabilities in multi-factor authentication and other advanced security mechanisms.
- Adaptive Threat Modeling: Tailors attack simulations to match an organization’s unique infrastructure.
- Behavioral Mimicry: Replicates human-like attacker behavior, exposing gaps in existing defenses.
Stat: AI-assisted phishing emails, created using Generative AI, show a 35% higher success rate due to their realism and personalization. (Source: Europol, 2023)
Example: Tools like DeepExploit leverage AI to craft adaptive payloads, uncovering vulnerabilities often missed by human testers.
Enhancing Penetration Testing
Generative AI transforms penetration testing workflows by automating repetitive tasks and improving accuracy:
- Automated Reconnaissance: Quickly maps network architectures using open-source intelligence (OSINT).
- Real-Time Guidance: Suggests step-by-step actions based on scan results.
- Comprehensive Coverage: Generates exhaustive test cases, ensuring no critical areas are overlooked.
Case Study: A penetration test using AI-driven tools identified misconfigurations in cloud services, allowing proactive remediation before exploitation. (Source: Hilario et al., 2024)
Addressing Challenges and Ethical Concerns
While Generative AI provides immense benefits, it also raises significant challenges:
- Over Reliance on AI: Human oversight is crucial to validate findings and address false positives or negatives.
- Bias in Data: AI models trained on flawed datasets can miss vulnerabilities or produce inaccurate results.
- Potential Misuse: Generative AI can be exploited by malicious actors to create advanced persistent threats (APTs) or polymorphic malware.
Stat: A 2023 BlackBerry survey revealed that 74% of IT decision-makers believe AI tools like ChatGPT are being used by nation-states for malicious purposes. (Source: CyberArk Research, 2023)
Organizations must adopt responsible AI practices, including rigorous testing, data privacy safeguards, and ethical oversight, to mitigate these risks.
Practical Applications for Cybersecurity Professionals
Generative AI tools cater to both seasoned experts and beginners:
- Beginner-Friendly Interfaces: Tools like Shell GPT simplify tasks, making them accessible to amateur testers.
- Advanced Features for Experts: Customizable scripts and real-time assessments empower experienced cybersecurity teams.
- Cross-Industry Applications: Sectors like healthcare and finance benefit from AI-driven vulnerability detection tailored to industry-specific challenges.
Stat: AI-driven systems, such as the DARPA Cyber Grand Challenge winner, showcased real-time vulnerability detection and patching, achieving results in minutes. (Source: DARPA, 2016)
Building a Resilient Cybersecurity Framework
Generative AI helps organizations create proactive defense strategies by:
- Predictive Analysis: Identifying trends in cyberattacks to prevent future breaches.
- Collaboration Platforms: Facilitating communication between security teams for cohesive strategies.
- Scalable Solutions: Adapts to growing networks and evolving threats seamlessly.
To Sum Up
Generative AI is transforming the way cybersecurity professionals identify vulnerabilities and mitigate risks. By detecting weaknesses, simulating innovative attack vectors, and enhancing penetration testing, it equips organizations to stay ahead of evolving threats. However, ethical concerns, data bias, and overreliance on AI necessitate responsible deployment and human oversight. Embracing Generative AI allows professionals to build resilient cybersecurity frameworks that protect against increasingly sophisticated cyberattacks.
References
- Hilario, E., et al. (2024). Generative AI for Pentesting: The Good, the Bad, the Ugly. International Journal of Information Security, 23, 2075–2097. https://doi.org/10.1007/s10207-024-00835-x
- OpenAI. (2023). ChatGPT and Large Language Models for Cybersecurity Applications. Retrieved from https://openai.com.
- CyberArk Research. (2023). Polymorphic Malware and AI-Driven Threats. Retrieved from https://cyberark.com/research.
- Europol. (2023). AI and Cybercrime: Risks and Preventive Measures. Retrieved from https://europol.europa.eu.
- DARPA. (2016). Cyber Grand Challenge: Autonomous Cyber Operations. Retrieved from https://www.darpa.mil.