Deep Learning Methods for Deepfake Detection – A Comprehensive Review
Share
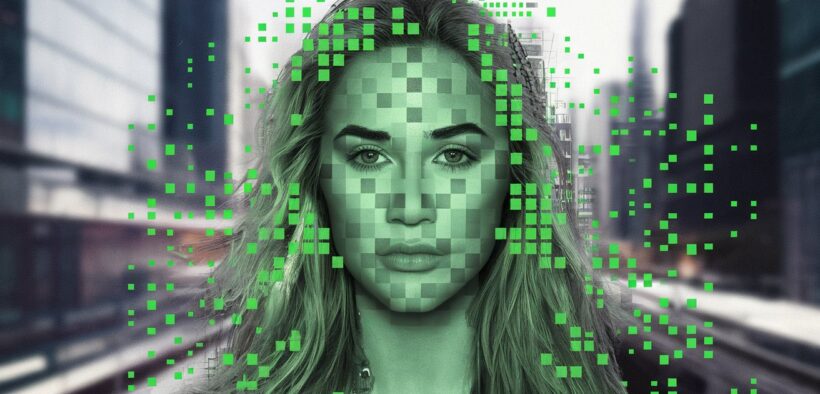
The advent of deep learning has transformed various industries, from healthcare to entertainment. However, with its immense benefits comes a dark side—deepfakes. These AI-generated images, videos, and audio recordings are designed to mimic real-life content with such precision that it becomes nearly impossible to distinguish between real and fake with the naked eye. For cybersecurity professionals and those aspiring to enter this field, understanding deepfakes and how they can be detected is essential to safeguarding truth and preventing the potential misuse of this technology.
What Are Deepfakes?
Deepfakes are synthetic media that use deep learning models, particularly neural networks, to create highly realistic forgeries of images, videos, and even audio. The name “deepfake” stems from “deep learning” and “fake,” emphasizing its reliance on sophisticated machine learning techniques to fabricate content. The widespread availability of tools and platforms that facilitate the creation of deepfakes has raised serious concerns about the misuse of this technology, from political misinformation to the creation of malicious content like revenge porn.
The Dangers of Deepfakes
Deepfakes pose significant threats to both individuals and institutions. From undermining public trust in media to influencing political events and legal processes, these AI-generated forgeries can have far-reaching consequences. Cybercriminals have already started exploiting deepfakes for identity theft, blackmail, and financial fraud, often targeting high-profile figures like politicians and celebrities. Additionally, deepfake-generated content could be used to destabilize governments or influence elections by impersonating political figures and spreading false information.
How Are Deepfakes Created?
Deepfakes are primarily created using two types of deep learning models: autoencoders and generative adversarial networks (GANs). In a GAN, two neural networks work together—a generator that creates fake content and a discriminator that attempts to identify whether the content is real or fake. Over time, the generator learns to create increasingly realistic fakes, leading to content that can deceive even the most discerning human eye. These techniques can be used for:
- Identity swap: Replacing a person’s face in a video with another’s.
- Attribute manipulation: Changing characteristics such as age, gender, or facial expressions.
- Expression swap: Altering the facial expression of a subject in a video to another.
Detecting Deepfakes
As deepfakes become more convincing, the challenge of detecting them has grown. Researchers have turned to the same technology—deep learning—that creates deepfakes to develop tools for detecting them. According to Heidari et al. (2023), convolutional neural networks (CNNs) are among the most commonly used methods in deepfake detection, particularly for video-based deepfakes. By training these networks on large datasets of real and fake content, they can learn to identify subtle differences between genuine and synthetic media.
Detection methods are typically divided into:
- Image detection: Analyzing single frames to identify manipulated content.
- Video detection: Focusing on inconsistencies in sequences of frames.
- Audio detection: Identifying fake audio by analyzing speech patterns and inconsistencies.
Popular Detection Techniques
- CNN-Based Detection: Deepfake detection often relies on CNNs, which are highly effective at extracting spatial features from images. CNN-based methods analyze individual frames of a video to identify discrepancies in texture, lighting, or facial features, which are often indicative of a deepfake. These networks are trained on vast datasets to recognize patterns that humans cannot easily detect, such as unnatural pixel arrangements or distorted facial structures.
- Hybrid Approaches: Hybrid detection models combine both image and audio analysis to improve accuracy. Since deepfakes are often used in multimedia contexts, analyzing the synchronization between video and audio streams can help identify inconsistencies. By detecting mismatches between lip movements and the corresponding audio, hybrid methods can effectively flag manipulated content.
- Region-Based Convolutional Neural Networks (RCNN): While CNNs focus on spatial data, RCNNs analyze both spatial and temporal data, making them more effective for video deepfake detection. RCNN models look at multiple frames within a video, capturing changes in facial expressions or subtle inconsistencies in the background across time.
The Challenges of Detection
Despite the progress made in detecting deepfakes, several challenges remain. One of the most significant hurdles is the rapid advancement of deepfake generation techniques. As detection algorithms improve, so do the methods for creating even more convincing fakes. Additionally, some deepfakes are designed with minimal manipulation, making it difficult for detection models to pick up on subtle changes. Another challenge is the availability of large datasets required for training deep learning models, which often struggle to generalize well to new or unseen content.
The Future of Deepfake Detection
Looking ahead, there are several areas where deepfake detection technology could improve. Heidari et al. highlight the need for better detection models that can handle audio, video, and images simultaneously. Additionally, there is a growing interest in leveraging transfer learning—where models trained on one dataset are fine-tuned for use in another domain—to improve the efficiency and accuracy of detection tools.
Another promising avenue is the integration of blockchain technology to enhance the authenticity of multimedia content. By verifying the origin and history of digital files, blockchain could help establish the provenance of media, making it harder for malicious actors to spread deepfakes without detection.
To Sum Up
The rise of deepfakes represents a significant challenge in the digital age. For cybersecurity professionals, staying ahead of this evolving threat is critical. By leveraging advanced deep learning techniques for detection, it is possible to combat the misuse of this technology and safeguard the integrity of digital content. However, as deepfakes become increasingly sophisticated, ongoing research and development in detection methods will be essential to ensuring a secure and trustworthy digital environment.
Reference article: Deepfake detection using deep learning methods: A systematic and comprehensive review – Heidari – 2024 – WIREs Data Mining and Knowledge Discovery – Wiley Online Library